In this month’s Spotlight, we are excited to highlight CADRE briefs and Learning Series recordings focused on aspects of artificial intelligence (AI) in STEM education and feature seven projects that are leveraging AI to improve teaching, learning, and research. The Spotlight also includes two blogs on the potential for AI to transform game-based learning, AI related publications from DRK-12 products, and a list of additional projects working in this area.
In this Spotlight:
- Artificial Intelligence in STEM Education | Briefs & Webinar Recordings
- Featured Projects
- An AI-Enhanced Colleague for Teachers: Developing and Studying an Innovative Platform for Efficient, Inclusive Middle-Grade Mathematics Lesson Planning (PI: Sun)
- Engaging High Schoolers in Integrated Computer Science and Engineering Through Hands-On Experiences with Microelectronics and Artificial Intelligence (PIs: Antonenko, Eutsler, Hoque)
- Evaluating Effects of Automatic Feedback Aligned to a Learning Progression to Promote Knowledge-In-Use (PI: Haudek)
- Intelligent Science Stations: Developing Adaptive Mixed-Reality Technology to Enhance Inquiry-based STEM Learning in Schools (PI: Yannier)
- Interactive, Individualized Professional Learning for Elementary School Teachers: Enhancing Content and Pedagogical Content Knowledge as a Basis for Improving Practice (PI: Copur-Gencturk)
- Supporting Instructional Decision Making: The Potential of Automatically Scored Three-Dimensional Assessment System (PIs: Harris, Krajcik, Yin, Zhai)
- Using Natural Language Processing to Inform Science Instruction (PIs: Linn, Liu)
- Additional Projects
- Related Resources
Artificial Intelligence in STEM Education | Briefs & Webinar Recordings
Briefs on AI in STEM Education
This three-part series of briefs were inspired by the question, “What are the essential considerations for researchers and developers who are
designing, studying, and using AI in K–12 STEM classrooms?”
Toward Ethical and Just AI in Education Research
Authors:
The first brief of the series offers guidance to researchers and developers for responsible AI research and implementation in educational settings. The brief describes an ethical artificial intelligence in education (AIED) framework and a set of tools to support inclusive and equitable AIED research and development.
Generative AI in STEM Teaching: Opportunities and Tradeoffs
Authors: Jeremy Price and Shuchi Grover
The second brief of the series examines how GenAI may shape STEM classrooms in the near future and identify promising trajectories for integrating GenAI into STEM teaching, including the potential for personalized teaching approaches. They reaffirm the essential role of teachers and emphasize the need to include teachers in the design, development, and study of GenAI tools that are intended to benefit STEM education.
The Potential of Using AI to Improve Student Learning in STEM: Now and in the Future
Authors: Namsoo Shin, Kevin Haudek, and Joseph Krajcik
The third brief explores how artificial intelligence (AI) can serve as a transformative learning partner in K–12 STEM education through AI's potential to offer personalized support, scaffold cognitive and metacognitive processes, and enhance student motivation and collaboration. Additionally, the authors consider how AI can promote access to STEM learning, while also addressing the need to identify and mitigate biases in AI system development to ensure broad and effective application.
CADRE Learning Series
In a related CADRE Learning Series, we heard from the authors of the briefs described above and engaged in discussions on the Ethical Use of AI in STEM Education Research and the potential of Generative AI in STEM Teaching and Learning: Now and in the Future. View the recordings and slides.
An AI-Enhanced Colleague for Teachers: Developing and Studying an Innovative Platform for Efficient, Inclusive Middle-Grade Mathematics Lesson Planning
PI: Min Sun | Co-PIs: Wei Ai, Rachel Ayieko, Jing Liu, Lorraine Males
Disciplines: Mathematics
Grade Levels: 6-8
Role of AI: Supporting Research/Analyses, STEM Teaching, STEM Learning, Other (see below)
This project is developing Colleague AI, a platform to streamline lesson planning and assist teachers in creating high-quality lessons as Colleague AI integrates AI tools across STEM education. In teaching support, the platform provides intelligent lesson planning, differentiation suggestions and incorporates a nudging chatbot to guide teachers to iteratively refine their lessons. Learning enhancement is achieved through high-quality lesson material generation, inclusive practices, and interactive problem-solving guidance. The enhanced lesson materials are expected to lead to improved instructional delivery and student mathematical learning outcomes. Rather than replacing teacher creativity, Colleague AI serves as a collaborative tool to enhance professional practice while reducing the time burden of lesson preparation.
AI Tools: Colleague AI develops domain-specific AI algorithms that integrate educational expertise with advanced AI technologies including semantic search, recommender systems, nudging chatbots, and multimodal content generation. These custom tools are specifically designed for mathematics education, with development guided by math educators and researchers to ensure accuracy and trustworthiness. The platform's AI tools focus on transforming vetted, open-access lesson plans into adaptable, high-quality instructional materials.
Project Description: Colleague AI is creating an innovative educational platform that focuses on transforming middle-grade mathematics instruction by integrating artificial intelligence into the lesson planning process. The project aims to enhance teacher productivity and reduce workload stress while ensuring high-quality, inclusive mathematical instruction for grades 6-8. Through AI-powered tools like Claire AI, developed with mathematics educators and researchers, we support teachers by streamlining lesson planning and helping teachers adapt instructional materials to meet diverse student needs.
The platform leverages semantic search, recommender systems, and content generation capabilities to help teachers modify and create engaging mathematics lessons. By focusing specifically on middle-grade mathematics, where content complexity increases and teacher support is crucial, Colleague AI helps ensure materials maintain mathematical accuracy while supporting different learning styles and ability levels.
This integration of educational expertise with AI technology aims to address the unique challenges of middle-grade mathematics instruction. The platform supports teachers in creating research-based, standards-aligned lessons while reducing preparation time, particularly benefiting early-career teachers and those serving diverse student populations. Through this focused approach, Colleague AI works to improve both teaching effectiveness and student mathematical achievement.
Challenges Related to AI Use in Education and Strategies for Addressing Them: In our experience implementing AI solutions in middle-grade math education, two significant challenges emerge in applying AI to mathematics education. First, general-purpose AI tools often generate lesson materials that lack conceptual depth, contain mathematical inaccuracies, and fail to reflect research-based teaching practices. We address this through developing domain-specific AI algorithms that integrate proven mathematical frameworks and are guided by mathematics educators' expertise, ensuring content maintains both academic rigor and pedagogical effectiveness. Second, while AI can streamline lesson planning, it's crucial to enhance rather than replace teacher creativity and professional judgment, especially when adapting materials for diverse student needs. Our solution involves implementing quality measures and tooling that supports teachers in iteratively refining AI-generated content, ensuring they maintain creative control while benefiting from AI assistance.
Initial Findings: Our initial implementation studies of Colleague AI's tools in math education have yielded promising preliminary results. Early qualitative data show teachers find Colleague AI generated lesson plans to be much more education-focused and responsive to specific instructional needs than generic LLM-based applications. One teacher found “with this tool, the generated lesson plan doesn’t need a whole rewrite - it’s only modification of small pieces.” Educators have observed substantial improvement in the efficiency and perceived efficacy of their lesson planning assisted by Colleague AI. The real time interactive capabilities of the platform allow teachers to iterate and refine the lesson plans much more efficiently; one teacher noted “my math expertise now matters ... AI generates an engaging math activity, giving me a starting point. It literally takes seconds to generate, rather than the 30 or 40 minutes it would have taken me to design the activity myself.”
When comparing human expert generated lesson plans to an early version of Colleague AI generated plans in middle grades, teacher raters had a slight overall preference for human designed lesson plans, but educators are increasingly accepting AI-generated lesson plans that leverage subject-specific training data and pedagogical expertise.
Products:
- Project Website: Practitioners can sign up and try the colleague.ai platform
- Papers & Publications
- Nucci, D., Liu, A., Sun, M., & Males, L. (2024). The professional knowledge required for high-quality AI-generated mathematics lesson planning. AMTE Connections. https://amte.net/sites/amte.net/files/Nuccietal_Connections_Fall2024.pdf
- Sarkar, S., Liu, A., Shapiro, B. R., & Sun, M. (2024). Collaborative design for educational AI: Empowering educators as co-creators. Working paper. https://www.amplifylearn.ai/wp-content/uploads/2025/01/isls_arxiv.pdf
- Liu, A., & Sun, M. (2023). From voices to validity: Leveraging large language models (LLMs) for textual analysis of policy stakeholder interviews. arXiv preprint arXiv:2312.01202. https://www.amplifylearn.ai/wp-content/uploads/2023/12/2312.01202.pdf
Engaging High Schoolers in Integrated Computer Science and Engineering Through Hands-On Experiences with Microelectronics and Artificial Intelligence
PI: Pavlo Antonenko, Lauren Eutsler, Tamzidul Hoque | Co-PIs: Swarup Bhunia, Andrea Ramirez-Salgado
Disciplines: Computer engineering, computer science, data science
Grade Levels: 9-12
Role of AI: Supporting Research/Analyses, Teaching and Learning Integrated Computer science and Engineering
Description: Our three-year, Level II, Design and Development DRK-12 project in the Learning Strand aims to broaden access to microelectronics and AI education focusing on altruistic engineering design to support high-schoolers’ interest and self-efficacy in integrated computer science and engineering. We are leveraging a low-cost embedded system (the AHA! board) and edge intelligence (Artificial Intelligence performed on local devices rather than in the cloud) to engage students and teachers from under-resourced communities in the design and creative application of smart, embedded technologies while scaffolding their engineering identity development to prepare them for the STEM jobs of tomorrow. Specifically, we are using Tiny Machine Learning (TinyML) to teach microelectronics and AI as it runs on stand-alone, low-cost microcontroller boards with extensive hardware abstraction, minimizing the barrier of integrating ML applications with hardware. Important to our approach, TinyML applications are robust enough to be used as solutions to real-world problems such as monitoring of local air quality, traffic safety, or physiology and health. Designing, deploying, and managing TinyML applications on microcontrollers exposes students to full-stack development skills that will be important both in college and in their future jobs because these skills apply to working with large-scale ML technologies and applications. Because TinyML does not require large amounts of data and model training employs straightforward procedures, this approach is accessible to students with low prior knowledge of ML, expanding their conceptions of AI to include hardware and sensor-based applications and illustrating responsible and ethical uses of AI.
Challenges Related to AI Use in Education and Strategies for Addressing Them: Access to affordable hardware, software, and datasets for demystifying integration of computer science and engineering, and the applications of AI beyond chatbots and LLMs. Solution: We have created an innovative embedded system that integrates 6 sensors and an inexpensive but powerful microcontroller to design and deploy community-relevant edge AI applications. Students learn about computer science, microelectronics engineering, and edge intelligence in an integrated way.
Approahces to Partnership, Project Design, and/or Collaboration: We are co-designing and testing the curriculum as a research-practice partnership with 8 high school computer science and engineering teachers from FL, TX and KS.
Opportunities for AI Use in STEM Education Research: Educators and educational scholars should expand the focus of AI education beyond NLP and LLM focused applications such as chatbots and integrate hardware-focused AI approaches such as edge intelligence or computer vision-focused approaches such as image classification.
Advice for Others Considering AI Use in STEM Education Research: Our main advice is to focus more on the concepts and applications and less on the procedural aspects of the implementation. This approach will help create and sustain situational interest among students.
Initial Findings: Currently in our initial project phase, we began co-designing the AHA! curriculum. Our five-module curriculum is intended to be taught in a flexible manner over 15 hours of instructional time. It leverages experiential hands-on learning using large coded datasets. We also constructed a more expansive and affordable AHA! Board. Our planning process includes brainstorming to create datasets to engage users with using multimodal sensing and components of the AHA! Board to solve real-world problems for social good (e.g., motion sensing, heart-rate monitoring).
Products:
- Ramirez-Salgado, A., & Antonenko, P. (2024, June). Exploring Outcome Expectations in Artificial Intelligence and Internet of Things in First-Year Engineering Students. Paper presented at 2024 ASEE Annual Conference & Exposition, Portland, Oregon. 10.18260/1-2--47422
- Ramirez-Salgado, A., & Hossain, T., & Bhunia, S., & Antonenko, P. (2024, June), Board 393: Supporting Hardware Engineering Career Choice in First-Year Engineering Students. Paper presented at 2024 ASEE Annual Conference & Exposition, Portland, Oregon. 10.18260/1-2--46979
Suggested Resources:
- EdX course: Foundations of TinyML
- Professional Certificate in Tiny Machine Learning (TinyML)
- Introduction to Embedded Systems Software and Development Environments
- LinkedIn Learning course: Edge AI: Tools and Best Practices for Building AI Applications at the Edge
Evaluating Effects of Automatic Feedback Aligned to a Learning Progression to Promote Knowledge-In-Use
PI: Kevin Haudek | Co-PIs: Leonora Kaldaras, Joseph Krajcik
Disciplines: Science
Grade Levels: 9-12
Role of AI: Supporting STEM Learning
AI Tools: Natural language processing, generative artificial intelligence, supervised machine learning
Project Description: This project develops and explores the impact of an artificial intelligence (AI) assessment system that automatically generates feedback based on students' open-ended responses in physical science, aligned with established learning progressions on electrical interactions. Learning progressions are cognitive models that are based on a developmental approach to learning. The AI scoring system will offer cognitive, tailored feedback to students based on their responses and provide teachers with class summaries. Such tools support formative assessment within high school physical science that meets the Next Generation Science Standards' performance expectations, aimed at enhancing student learning.
The AI system uses natural language processing, image recognition, and supervised machine learning to evaluate students' written explanations and digitally created models. The feedback is tailored to the learning progression and delivered according to the AI's classification of the responses. The study assesses whether this automated feedback enhances students' learning outcomes and their progression regarding electrical interactions. Additionally, the project fosters scientific knowledge by involving students in scientific practices like modeling, connecting core disciplinary concepts, and using crosscutting ideas to analyze compelling phenomena, with real-time feedback provided throughout the process.
Challenges Related to AI Use in Education and Strategies for Addressing Them: One of the important challenges we have faced in the current project is simultaneous analysis of student performance on multi-modal assessments aligned to a knowledge-in-use LP. An example of an LP-aligned multi-modal assessment item is asking students to develop a scientific model explaining an electrostatic phenomenon and write a short accompanying explanation reflecting their reasoning. Such assessments are challenging to evaluate using AI due to the need to simultaneously evaluate the model, the explanation, and make a decision on final LP-level placement. Moreover, the resulting feedback should provide productive and constructive comments to specific ideas present in the student response in order to support the student developing more sophisticated understanding as described by the LP. In the current project, we have evaluated the two modalities separately using AI, and then humans make decisions regarding the final LP level placement and feedback based on the two modalities. In the future, we hope to explore multimodal AI analysis, as this will better align with the assessment tasks for knowledge-in-use.
Initial Findings: We have designed and tested a learning progression guided approach to training AI algorithms to evaluate complex science understanding reflective of knowledge-in-use in different modalities: text-based explanations and drawing-based scientific models. Preliminary results indicate that this approach helps develop rubrics for human and AI-based scoring that yield high human-human and human-AI agreement on both modalities. These results suggest promise in training AI algorithms to evaluate complex reasoning and skills in a way that is grounded in relevant, empirically validated learning theories, reflecting human-in-the loop approach to AI training.
Products:
- Project Website
- Presentations & Publications
- Kaldaras, L., Li, T., Haudek, K., & Krajcik, J. (2024, April 12). Developing rubrics for AI scoring of NGSS (Next Generation Science Standards) learning progression–based scientific models. Paper presented at the 2024 Annual Meeting of the American Educational Research Association. doi: 10.3102/2109181
- Li, T., Kaldaras, L., Haudek, K., & Krajcik, J. (2024, April 11). Using AI to evaluate multimodal formative assessments in physical science. Poster presented at the 2024 Annual Meeting of the American Educational Research Association. doi: 10.3102/IP.24.2112964
- Kaldaras, L., Haudek, K., & Krajcik, J. (2024). Employing automatic analysis tools aligned to learning progressions to assess knowledge application and support learning in STEM. International Journal of STEM Education, 11(1), 57. https://doi.org/10.1186/s40594-024-00516-0
Intelligent Science Stations: Developing Adaptive Mixed-Reality Technology to Enhance Inquiry-based STEM Learning in Schools
PI: Nesra Yannier | Co-PIs: Scott Hudson, Ken Koedinger
Disciplines: Science
Grade Levels: PreK-5
Role of AI: Supporting STEM Learning
AI Tools: We have a patented AI and mixed-reality system that uses AI computer vision to track what children are doing in the real world as they do scientific experiments and provides personalized, adaptive, interactive feedback.
Project Description: We have been developing and researching Intelligent Science Stations, a new genre of interactive science experiences that add an AI layer on top of physical experimentation to improve children’s STEM learning and engagement. The motivation behind this project is the need for engaging, inquiry-based science learning opportunities for young children in the classroom, to sustain early interest in science. The Intelligent Science Stations provides students with hands-on science experiences, augmented by an intelligent agent that offers feedback based on artificial intelligence (AI) computer vision. These Intelligent Science Stations incorporate scientific apparatuses such as earthquake tables, ramps or balance scales, and students' actions are observed and evaluated by the intelligent agent. The agent appears as an animated character on a screen and provides interactive feedback and guidance to guide students through scientific inquiry. This innovative approach offers evidence-based, personalized support and feedback to children, while also assisting teachers in integrating more inquiry-based science learning into their classrooms. By modeling behaviors like asking questions, making predictions, and explaining scientific phenomena, the interactive AI system helps teachers enhance their classroom experiences.
Challenges Related to AI Use in STEM Education and Strategies for Addressing Them: Based on our research, we have seen that many teachers, especially in elementary school, don’t have a science background so are afraid to teach science concepts. They want technologies that support them together with the children. Here is what one of the teachers said after seeing our system: “I love that NoRILLA uses technology in such an engaging, communicative and non-isolating way. I'm not a scientist, I'm not a scientist by any stretch of imagination and I love science and I love to teach science, but I feel like I'm limited by own limitations in the science world. To have something like this that supports and backs up and lets the kids and myself all learn together is genius!"
Approaches to Partnership, Project Design, and/or Collaboration: We believe good partnerships and diverse perspectives are instrumental to success. We try to partner with many organizations from different perspectives and we prioritize their needs to make sure our technologies and curriculum can be integrated in the real-world seamlessly.
Opportunities for AI Use in STEM Education Research: I believe advanced technologies and AI have great potential to improve children's learning and engagement in the classroom if used in the right way. I think it's important to use technology in a creative way to get children to think critically rather than memorizing facts or pressing buttons.
Advice for Others Considering AI Use in STEM Education Research: I think it's important to leverage AI in a way that's not isolating but instead more collaborative, in a way that brings people together rather than bringing them apart. It's also crucial to have an evidence-based approach to build technologies that are scientifically proven to be effective in the real-world.
Initial Findings: Early research has shown that AI-enhanced intelligent science stations improve children’s STEM learning by 5 times compared to other screen-based technologies. Also, adding an AI-based guided inquiry layer on top of physical experimentation improves children’s learning and engagement dramatically compared to traditional maker spaces and hands-on exhibits (4x dwell time).
Our recent research in schools has shown that children’s informed decision making and deep understanding of standards-aligned STEM concepts doubled in one year using NoRILLA Intelligent Science Stations. We also saw that there was a 32% increase in students’ ability to Explain their answers, which relates to Higher Level Thinking as highlighted in NGSS and common core standards. Looking at grades separately, we saw that a kindergartner interacting with NoRILLA for 3 months significantly exceeded the scientific understanding of a second grader (3 times the natural yearly progression).
Products:
- Project Website
- Yannier, N., Hudson, S. E., Koedinger, K. R., Hirsh-Pasek, K., Golinkoff, R. M., Munakata, Y., ... & Brownell, S. E. (2021). Active learning:“ Hands-on” meets “minds-on”. Science, 374(6563), 26-30.
Suggested Reading:
- Yannier, N., Hudson, S. E., & Koedinger, K. R. (2020). Active learning is about more than hands-on: A mixed-reality AI system to support STEM education. International Journal of Artificial Intelligence in Education, 30, 74-96.
- Yannier, N., Crowley, K., Do, Y., Hudson, S. E., & Koedinger, K. R. (2022). Intelligent science exhibits: Transforming hands-on exhibits into mixed-reality learning experiences. Journal of the Learning Sciences, 31(3), 335-368.
Interactive, Individualized Professional Learning for Elementary School Teachers: Enhancing Content and Pedagogical Content Knowledge as a Basis for Improving Practice
PI: Yasemin Copur-Gencturk | Co-PIs: Ken Frank, Jiliang Tang
Disciplines: Mathematics
Grade Levels: Elementary school teachers and their students
Role of AI: Supporting STEM Teaching
AI Tools: We are developing a new AI tool to create a scalable, real-time and personalized professional development program that offers teachers interactive learning opportunities to enhance their knowledge of teaching numbers and operations. Unlike many generative AI tools, our approach uses AI to foster an active learning environment in which AI acts as a facilitator for teacher learning. This means that teachers construct their own understanding through engaging specially designed activities tailored to their needs while the AI analyzes their responses, provides feedback, and offers additional learning opportunities in areas where further support is needed.
Project Description: Students learn more mathematics when their teachers are knowledgeable about the content and how best to teach that content to a range of students. As a result, teachers, like other professionals, need continuing support and training to be most effective. Unfortunately, that training is not always available -- teachers may have scheduling constraints that limit their opportunities to access support, or there may not be enough experts in their district to support them. Therefore, this project will develop AI support for teachers’ mathematics instruction. Once developed and adapted to context, the AI can draw on expert knowledge to support teachers on their schedules Specifically, we are creating a content-focused, interactive, and scaled professional development program to facilitate teachers’ learning through mimicking human facilitators. The AI analyzes teachers’ responses, identifies areas that need further support, and provides follow-up learning opportunities, depending on the teachers’ needs.
Challenges Related to AI Use in STEM Education and Strategies for Addressing Them: The most significant challenge we face in this area is the limited incorporation of research on teaching and learning into the development of tools that leverage AI. The lack of attention to effective teaching and learning conditions in the design of these tools hinders their ability to promote meaningful learning, as they often replicate mistakes made in the past. For instance, these tools are typically designed to deliver information rather than provide opportunities to foster higher-order thinking skills. Our solution is to develop tools informed by existing research on what makes professional development effective for teachers and to find ways to design AI systems that support this goal.
Approaches to Partnership, Project Design, and/or Collaboration: Close collaboration between content and AI experts during the design phase of AI tools is essential for developing tools that have a meaningful impact on STEM teaching, learning, and research.
Opportunities for AI Use in STEM Education Research: One goal is to create accessible and personalized professional learning opportunities for teachers, with the potential to enhance teaching and learning on a large scale. Another significant implication lies in educational research because part of our aim is to enhance the ability of AI to understand the qualitative nuances in teachers’ knowledge and skills that only experts can detect. The advances we make in this area could be extended to automate data coding at scale, and enabling the capture of meaningful differences in teaching and learning. In fact, we are currently exploring the potential of AI in this regard: Can AI be trained to identify qualitative nuances in knowledge and skills as effectively as experts in the field?
Advice for Others Considering AI Use in STEM Education Research: We suggest focusing on the content being covered and the types of learning opportunities provided to build understanding. We have observed that some tools are not aligned with research on effective and meaningful STEM learning, which can potentially do more harm than good. We also recommend building an interdisciplinary team of computer scientists and math educators, each of whom knows how to speak the other’s language enough to pursue shared goals.
Initial Findings: We are currently identifying the foundational concepts central to numbers and operations, along with common learning patterns exhibited by students and evidence-based practices and representations that support deeper student understanding of these concepts. Additionally, we are exploring how to set clear learning goals for these activities so that AI can fully comprehend and leverage them for teachers’ professional development. Our efforts are focused on system design and developing ways for AI to capture the nuances in teachers’ responses. We are also working to identify accurate and efficient methods to assess teachers’ understanding.
Products: The prototype of our work, including how we develop the content for these programs and integrate educational technology, can be found here. It is important to note that this project leverages more advanced technologies, pushing the boundaries in both education and AI. Our efforts focus on creating interactive and personalized learning environments that foster the development of higher order thinking skills.
- Content Knowledge Identification with Multi-agent Large Language Models (LLMs)
- A LLM-Powered Automatic Grading Framework with Human-Level Guidelines Optimization
Suggested Reading:
- Improving Teaching at Scale: Can AI Be Incorporated Into Professional Development to Create Interactive, Personalized Learning for Teachers?
- The impact of an interactive, personalized computer-based teacher professional development program on student performance: A randomized controlled trial
- A promising approach to scaling up professional development: intelligent, interactive, virtual professional development with just-in-time feedback
Supporting Instructional Decision Making: The Potential of Automatically Scored Three-Dimensional Assessment System
PI: Christopher Harris, Joseph Krajcik, Yue Yin, Xiaoming Zhai | Co-PIs: David McKinney
Disciplines: Science
Grade Levels: Grades 6-8
Role of AI:
- Research/Analyses: AI is used to automatically score three-dimensional assessment items, providing timely scores for students' performance on the tasks.
- STEM Teaching: AI tools provide teachers with data-driven insights to enhance instructional decision-making.
- STEM Learning: Students benefit from personalized feedback generated by AI-based scoring systems, which helps identify strengths and areas for improvement.
AI Tools: Leveraging natural language processing (NLP) and large language models (LLMs) to score open-ended assessment item and integrating automated scoring systems with instructional dashboards for educators
Project Description: The PASTA (Providing Automated Scoring for Three-dimensional Assessments) project explores the potential of AI to revolutionize science education by leveraging automated scoring systems for three-dimensional assessments. These assessments align with the Next Generation Science Standards (NGSS) and focus on integrating disciplinary core ideas, scientific practices, and crosscutting concepts. Using advanced natural language processing techniques, the project has developed AI models that accurately and timely score open-ended student responses, providing teachers with actionable feedback.
By embedding these tools into user-friendly dashboards, the project empowers educators to make data-informed instructional decisions, ultimately enhancing the teaching and learning process. The PASTA project aims to bridge the gap between assessment and instruction by enabling real-time feedback, fostering equity in science education, and supporting differentiated instruction tailored to individual student needs.
Challenges Related to AI Use in STEM Education and Strategies for Addressing Them: One key challenge is managing unbalanced training data for developing automatic models. To address this, the project explored data augmentation strategies to enhance model performance. Another challenge involves the large size of LLMs, which consume costly resources. The project studied this issue by using knowledge distillation to reduce model size while maintaining effectiveness.
Approaches to Partnership, Project Design, and/or Collaboration: Collaborations with teachers, assessment experts, and AI researchers have been instrumental in designing tools that are both technically robust and pedagogically meaningful.
Opportunities for AI Use in STEM Education Research: The integration of AI-powered adaptive learning systems that provide personalized recommendations for instructional strategies based on assessment data.
Products & Initial Findings: The project is developing a dashboard interface and automated scoring platform. Reports, publications, and evaluation results can be accessed on our project page.
Suggested Reading: Zhai, X., & Nehm, R. H. (2023). AI and formative assessment: The train has left the station. Journal of Research in Science Teaching, 60(6), 1390-1398.
Using Natural Language Processing to Inform Science Instruction: Technologies to Inform Practices in Science (TIPS)
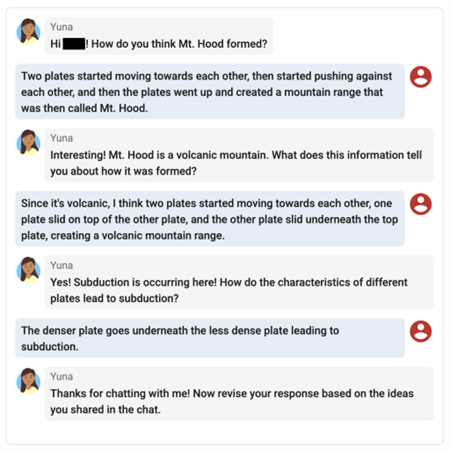
PIs: Marcia Linn, Lei Liu | Co-PIs: Libby Gerard, Quang Hoang
Disciplines: Earth Science, Life Science, Physical Science, Engineering
Grade Levels: 6-12
Role of AI: Supporting Research/Analyses, STEM Teaching, STEM Learning
AI Tools: Software Tools: TIPS uses NLP idea detection models to identify each idea in a student explanation. TIPS partners compose pedagogically informed prompts for each idea to guide the student to link their idea to a new idea. The TIPS Dialog uses the NLP to detect student ideas in real time and assigns one of the partner-authored prompts. Rather than only addressing frequent ideas, TIPS affirms and builds on each student’s ideas.
TIPS leverages industry-standard, open-source AI tools and frameworks, including Hugging Face, PyTorch, and Weights & Biases (W&B), to facilitate the development, monitoring, and training of our Natural Language Processing (NLP) neural network models. For deployment, we utilize robust cloud-based tools from Amazon Web Services (AWS) such as EC2 and SageMaker, ensuring scalable and efficient operationalization of our models.
TIPS AI Dialog. We exploit NLP to identify each student’s science ideas in their written explanation in response to a knowledge integration question about an NGSS topic. For example, one question in a unit on photosynthesis asks students to write a story about how animals use energy from the sun. Our NLP model can detect about 25 different student science ideas within their written responses. Students often express ideas both from instruction and ideas from their own experiences.
We designed an adaptive Dialog that uses the NLP idea detection and embedded the dialog in web-based inquiry science units. The tool uses knowledge integration pedagogy to engage the student in a personalized dialog to integrate their specific detected ideas about the topic. An avatar detects each student’s initial ideas, asks questions tailored to the student’s ideas, and helps the student generate a more coherent and accurate science explanation.
We designed the NLP idea detection models and dialog guidance in partnership with local middle and high school science teachers to ensure that the models are detecting the full range of student ideas they hear in the classroom. Our recent findings demonstrate that the chatbot can improve students’ science understanding. Students like the chatbot and want the conversation about their science ideas to continue. Check out recent findings below!
AI Peer Chat. We also utilize NLP idea detection technology in an online peer chat tool. We designed the tool in partnership with middle school teachers and have embedded it in two web-based inquiry science units such as Plate Tectonics and Photosynthesis. The tool pairs students so that they can chat about their ideas about a focal science topic. The peer chat tool uses the same NLP technology described above to recognize students’ ideas and adaptively pair them based on parameters set by researchers or teachers. For example, a recent study paired students to maximize the number of different ideas students expressed between them (Billings et al., 2024). Students can also be paired based on KI score using previously developed KI scoring NLP models. We are currently working on additional adaptive pairing strategies, including pairing students based on specific ideas they express in their explanations.
The peer chat also provides students with adaptive question bank prompts to help facilitate their online discussion. The prompts are assigned to student pairs based on the ideas detected in each of the student’s explanations using NLP. Students can use the question bank prompts in the chat environment to help facilitate and inspire their discussion. Findings from a recent study indicated that the adaptive question bank was used effectively by students and students were more likely to use the NLP adaptive prompts than generic prompts (Billings et al., 2024).
Project Description: Technologies to Inform Practices in Science (TIPS) uses AI to build integrated understanding by guiding students to elaborate their explanations of complex scientific phenomena. We implement peer conversations and adaptive dialogs that prompt students to make their explanations more coherent. We embed the AI dialogs in interactive, web-based units featuring models of the scientific topic, hands-on activities, and projects.
TIPS uses Natural Language Processing (NLP) idea detection models to identify each idea in a student explanation. The models meet industry standards for human-machine agreement. TIPS partners compose pedagogically informed prompts for each idea. The embedded dialog detects student ideas in real time and assigns one of the prompts (see Figure). Rather than only addressing frequent ideas, dialogs affirm and build on each student’s ideas, consistent with knowledge integration pedagogy.
To minimize possible bias in NLP idea detection towards Eurocentric culture, in partnership with teachers, we build the models using responses of students with similar demographics to student users in future science classes. The partner teachers’ schools serve a majority of students from underrepresented groups in STEM.
We show that AI can improve peer conversations and adaptive dialogs that promote students’ science understanding. Adding dialogs to inquiry science units, enables students to consider more and better ideas in their explanations and make more productive revisions to their thinking. The adaptive dialog engages students from both monolingual English and multilingual backgrounds in adding relevant ideas while revising their explanations. Students like participating in dialogs and want the conversation about their science ideas to continue.
Challenges Related to AI Use in STEM Education: Building educationally valid models
Approaches to Partnership, Project Design, and/or Collaboration: Research Practice Partnership where each partner respects the expertise of the other partners and commits to learn from each other.
Opportunities for AI Use in STEM Education Research: Personalizing interactions with students, informed by advances in pedagogy.
Advice for Others Considering AI Use in STEM Education Research: Build on the work of others, create and use open source materials. Connect innovation with powerful pedagogy to amplify impacts and outcomes.
Initial Findings: Our recent findings demonstrate that the AI dialog can improve students’ science understanding. Students like the dialog and want the conversation about their science ideas to continue. The adaptive dialog engages students from both monolingual English and multilingual backgrounds in incorporating additional relevant ideas into their explanations, resulting in a significant improvement in student responses from initial to revised explanations. The guidance supports students in both language groups to progress in integrating their scientific ideas. TIPS NLP models achieve industry-standard human-machine agreement.
Products:
- Video: 2022 STEM for All Video Showcase (Facilitators' Choice Award)
- Paper: Gerard, L., Holtman, M., Riordan, B., & Linn, M. C. (2025). Impact of an adaptive dialog that uses natural language processing to detect students’ ideas and guide knowledge integration. Journal of Educational Psychology, 117(1), 63–87. https://doi.org/10.1037/edu0000902
- WISE units with an AI dialog or peer chat:
- Additional Select Papers:
- Holtman, M., Gerard, L., Li, W., Linn, M.C., Steimel, K., & Riordan, B. (2023). How does an adaptive dialog based on natural language processing impact students from distinct language backgrounds? Proceedings of the International Society of the Learning Sciences (ISLS). Montreal, Canada: International Society of the Learning Sciences. https://repository.isls.org/handle/1/9918
- Riordan, B., Bichler, S., Bradford, A., King Chen, J., Wiley, K., Gerard, L., & Linn, M.C. (2020). An empirical investigation of neural methods for content scoring of science explanations. Proceedings of the Fifteenth Workshop on Innovative Use of NLP for Building Educational Applications, Association for Computational Linguistics, Seattle, WA. https://aclanthology.org/2020.bea-1.13/
- Billings, K., Chang, H-Y., Brietbart, J., & Linn, M.C. (2024). Using Artificial Intelligence to Support Peer-to-Peer Discussions in Science Classrooms. Education Sciences, 14(12). https://www.mdpi.com/2227-7102/14/12/1411
- Gerard, L., Bradford, A., Wiley, K., Debarger, A., & Linn, M.C. (May/June 2022). Cultivating teacher efficacy for social justice in science. Science Scope, 45(5), 35-43. https://www.nsta.org/science-scope/science-scope-mayjune-2022/cultivating-teacher-efficacy-social-justice-science
Additional Projects
We invite you to explore a sample of the other recently awarded and active work with a focus on AI in STEM education research.
- Advancing AI in Science Education (AASE): A Comprehensive Approach to Equity, Inclusion, and Three-Dimensional Learning (PI: Zhai)
- AI-based Assessment in STEM Education Conference (PI: Zhai)
- Capturing and Leveraging Data from Teacher-Student Interactions to Improve STEM Learning: An Incubator Project (PI: Reich)
- Designing Interactive Visualizations of Neural Pathways in Language-based AI for Secondary Students to Explore Interpretability of AI and Human-Machine Collaboration (PI: Chao)
- Developing Learning Trajectories Supporting Middle School Student Understanding of Artificial Intelligence Concepts (PI: Lee)
- Incubating the Use of Artificial Intelligence for Conducting High-Quality Research Syntheses (PI: Polanin)
- Investigation of Students' Learning, Interest, and Career Aspirations in an Integrated Science and Artificial Intelligence Learning Environment (i-SAIL) (PI: Akram)
- Learning Probability Through AI Problem-Solving in a Game-based Environment (PIs: Greenwald, Wang)
- A Semiconductor Curriculum and Learning Framework for High-Schoolers Using Artificial Intelligence, Game Modules, and Hands-on Experiences (PI: Lipsmeyer)
- Strengthening and Developing Partnerships in East Tennessee for Community Engagement in Artificial Intelligence Education (PI: Wong)
- Supporting Secondary Students' Earth Science Knowledge and Engineering Design Skills with Mobile Design Studios (PI: Schimpf)
Related Resources
- Additional DRK-12 Publications in the CADRE Library:
- The Effect of Automated Feedback on Revision Behavior and Learning Gains in Formative Assessment of Scientific Argument Writing
This study focuses on the latter and investigates a formative feedback system integrated into an online science curriculum module teaching climate change. The feedback system incorporates automated scoring technologies to support students' revision of scientific arguments. - Guiding Collaborative Revision of Science Explanations
This paper illustrates how the combination of teacher and computer guidance can strengthen collaborative revision and identifies opportunities for teacher guidance in a computer-supported collaborative learning environment. - Myths, Mis- and Preconceptions of Artificial Intelligence: A Review of the Literature
To develop effective educational programs in the field of AI, it is vital to examine and understand learners' pre- and misconceptions as well as myths about AI. This study examined a corpus of 591 studies. - Use of Automated Scoring and Feedback in Online Interactive Earth Science Tasks
In this study, we analyze log data to examine the granularity of students’ interactions with automated scores and feedback and investigate the association between various students’ behaviors and their science performance. - Using Artificial Intelligence Teaching Assistants to Guide Students in Solar Energy Engineering Design
We present Aladdin, a web-based computer-aided design (CAD) platform for engineering design with a built-in artificial intelligence teaching assistant (AITA). We also present two curriculum units (Solar Energy Science and Solar Farm Design), where students explore the Sun-Earth relationship and optimize the energy output and yearly profit of a solar farm with the help of the AITA. We tested the software and curriculum units with over 100 students in two Midwestern high schools.
- The Effect of Automated Feedback on Revision Behavior and Learning Gains in Formative Assessment of Scientific Argument Writing
- Community Voices Blogs:
- Artificial Intelligence, Learning Games, and the Potential to Connect, Engage, and Support STEM Learners | Eric Klopfer
- Using AI to Connect Game-Based and Narrative-Centered Learning | Jeremy Roschelle, Gautam Biswas, Cindy Hmelo-Silver, James Lester
- Related Spotlights: